Kafka Connect#
Kafka Connect is a tool used to integrate Kafka against other systems, in real time. Completely configuration-based, which can perform lightweight transformations on data. The system is fully de-coupled from the Kafka system.
Sources and Sinks#
Kafka Connects contains several connectors. A source connector imports data from an external system into Kafka, while a sink connector exports from Kafka to an external system.
Kafka Connect Components#
Kafka Connect is composed of several components:
Connector: Defines the interaction between Kafka Connect and external technology
Converter: Handles serializaiton and de-serialization of data, and plays a role in persistence of schemas
Transformer: Optional components that perform lightweight transformations on the data, as it flows though Kafka Connect. Single message transformations (SMT).
Using Kafka Connect#
Creating and managin connectirs is performed though a REST API. The connector implementation depends on the application,
and is configured though a connector.class
field. An example of creating a sink connector from Kafka to a file could
be as follows:
curl -X POST http://localhost:8083/connectors \
-header 'Accept: */*' \
-header 'Content-Type: application/json' \
-data '{
"name": "file_sink_connector",
"config": {
"connector.class": "org.apache.kafka.connect.file.FileStreamSinkConnector",
"topics": "connect_topic",
"file": "/home/cloud_user/output.txt",
"value.converter": "org.apache.kafka.connect.storage.StringConverter"
}
}'
Deploying Kafka Connect#
Deploying Kafka Connect only applies if we’re managing self-managed Kafka! Kafka Connect runs under JVM as a process known as worker, which can execute multiple connectors. Tasks are executed by workers, which are either standalone or distributed.
Distributed Workers#
Kafka Connect uses Kafka Topics to store state pretaining to connector config, status, ect. The topics are configured to retain this information indefinetly – compacted topics. Connector instances are created and managed using the REST API that Kafka Connect offers. Tasks are rebalanced to redistritubet the wrokload automatically, and crashed units will lead to a new rebalancing. Essentially, a Kafka Connect Cluster consists of one or multiple workers (preferably two or more), where the workers are responsbible for separate tasks. See figure below.
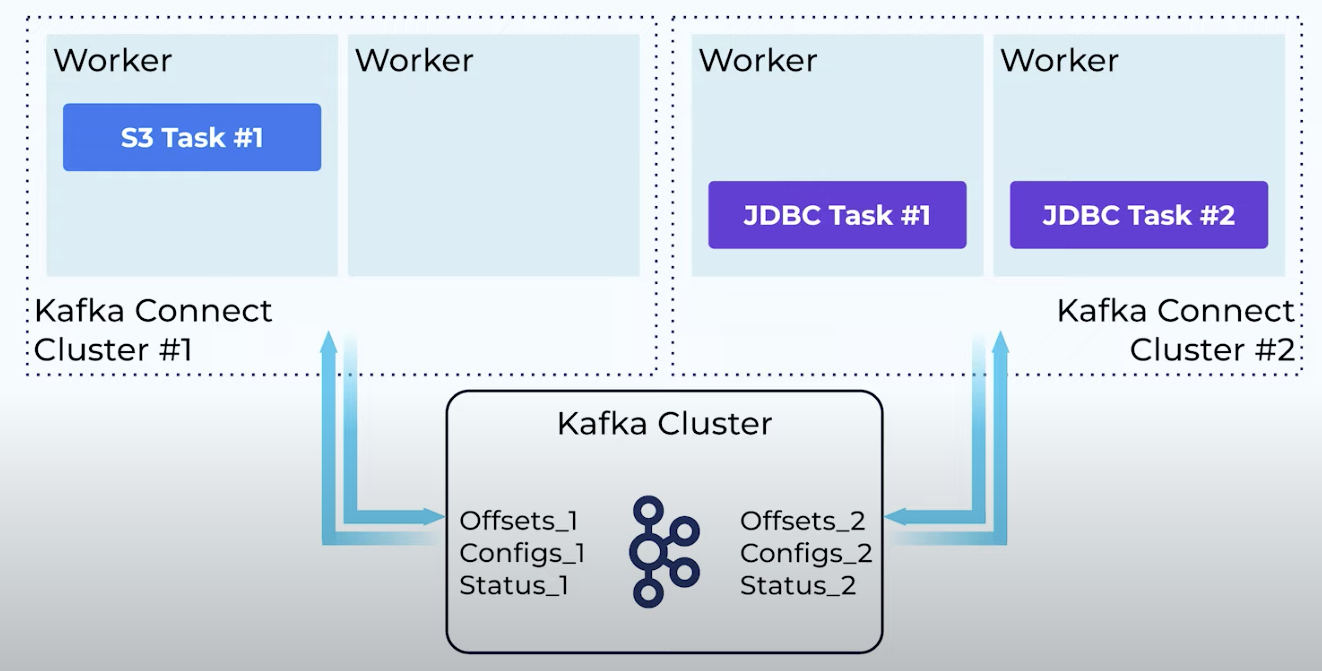
Fig. 5 Multiple workers on multiple clusters in a Kafka Connect Cluster.#
Standalone Workers#
In standalone mode, the worker uses files to store its state, not using the REST API. Cannot have fault-tolerant behavior or scale. Can include tasks such as reading from files from a particular machine (server locallity). This can also be performed using a distributed methodology.

Fig. 6 Multiple tasks on a standalong worker in Kafka Connect.#
Running Kafka Connect in Docker#
A Kafka Connect can be run in a container such as Docker or Kubernetes, though the image confluentinc/cp-kafka-connect
. Adding dependencies at runtime means no need to create new image, but will increase installation time. Containerized
solution not ideal for production.
Using the Kafka Connect API#
Perform a curl
call to get the basic information. Futhermore, plugins can be inspected by running:
curl -s localhost:8083/connector-plugins | jq '.'
To create a connector instance, PUT
or POST
a JSON file with connector configuration:
curl -i -X PUT -H <header-info> ect.
and similarly GET
configuration for an existing connector:
curl -i -X GET -H <header-info> ect.
You can also pause, restart, and resume a connector or specific task on a connector. Pausing a connected does pause the tasts.
Error handling#
Can be handled using different patterns: Using an inconvert converter can trigger an error: “Unknown magic byte!”. Another scenario is when a topic contains several differently serialized messages (e.g. Avro and JSON).
Fail fast: Default method; task fails and process stops.
Silent ignore:
Dead letter queue:A dead letter queue is a queue/message where failed messages are written to. Not configured automatically
From the dead letter queue (DLQ), the errored messages may be sent into another converter in case the schema is different. See figure.
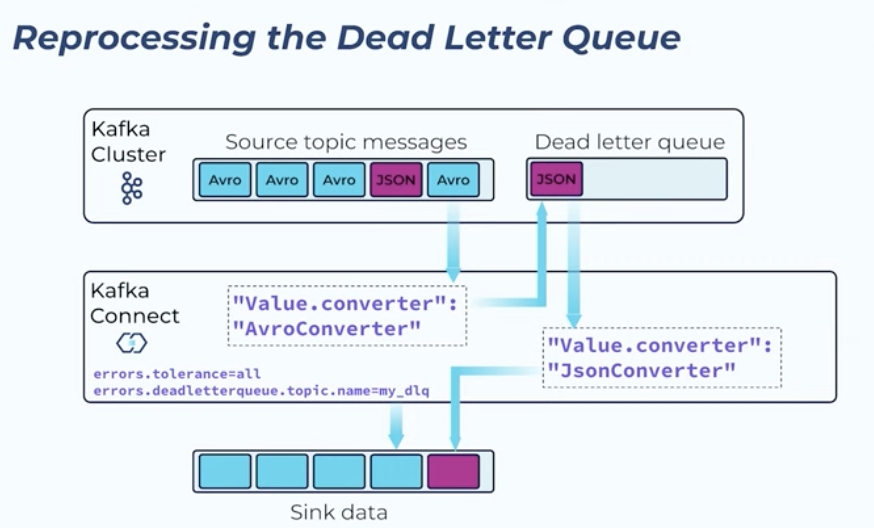
Fig. 7 Dead letter queue way of handling errors when serialization fails.#
DLQ is not default because it requires setup. What should happen to the DLQ messages; this needs to be predefined.